Building Your AI Analytics App from Scratch
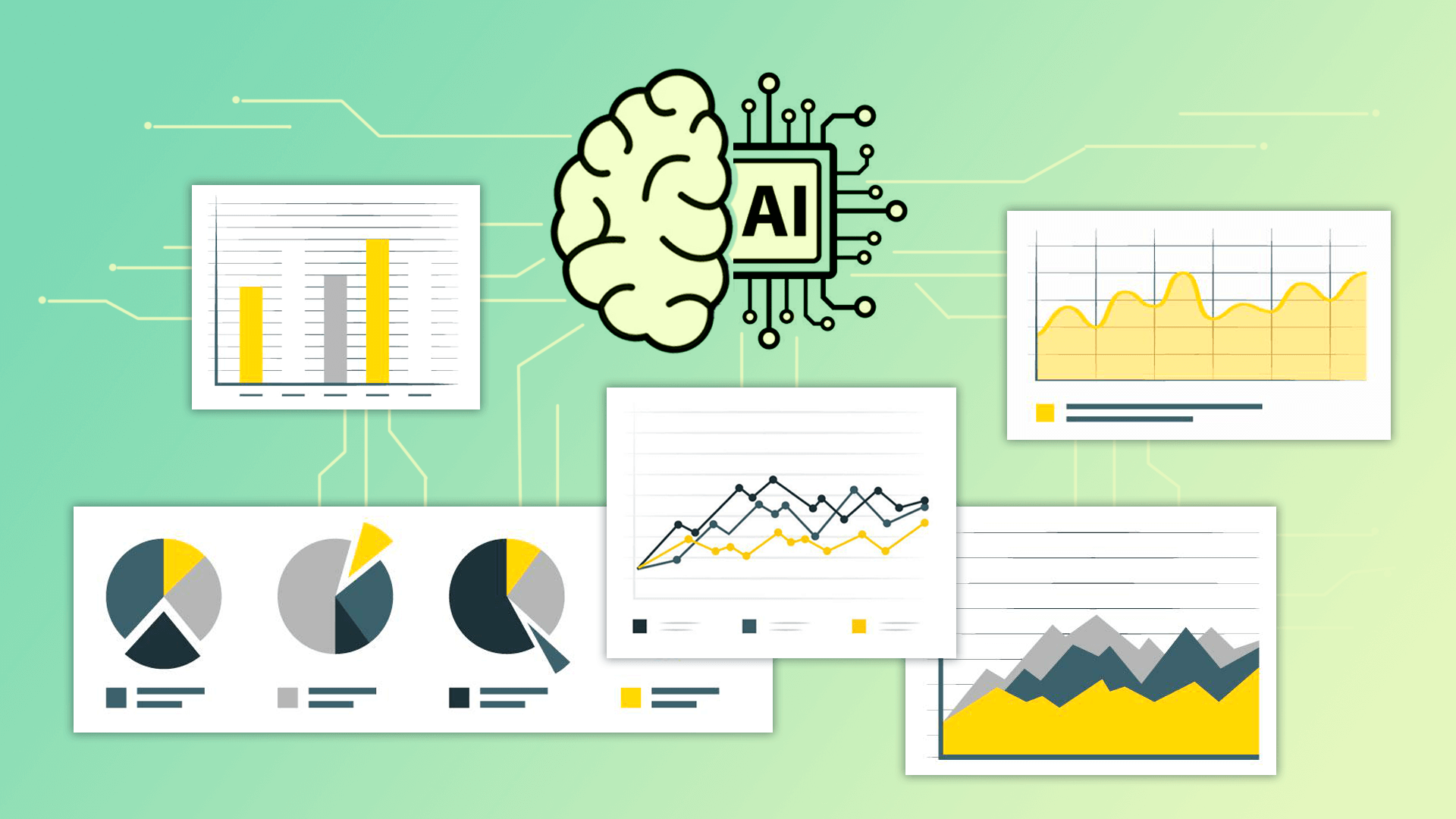
In its transformative journey, AI has come to change data collection and analytics processes at its core, providing groundbreaking possibilities for gathering and working with data.
AI analytics applications will have a very significant and special place in business processes, providing uninterrupted data analysis and scalability that were once impossible with existing tools and human potential. More and more businesses will invest in top-notch AI analytics applications that will help them get a big picture of customer behavior, customer relationships, their motivation behind decision-making, and more.
Here are some statistics about data analytics.
- 36% of businesses claim that data analytics have improved business processes and resulted in increased revenue.
- 94% of companies admit that data analytics are critical for digital transformation.
- 57% of companies already use data to build new strategies.
Understanding the importance of data in business processes, Addevice has leveraged the latest AI technologies to build AI analytics applications first for internal use and later to share with partners.
For now, let’s talk about data, AI, and how these two work together productively.
Understanding AI Analytics Applications
Before delving into the core processes, let’s first understand AI analytics. It is a subset of business intelligence (or soon to be a technology to replace BI) that processes large amounts of data with machine learning and without human input. In simple words, AI analytics automates most of the work of data analysts, but, of course, we aim to deliver more than automation.
AI analytics applications offer a modern measurement of understanding and premonition with a unused level of speed and granularity. Not at all like conventional analytics, which center on review investigation, AI analytics offer prescient experiences, prescriptive activities, and computerization, extricating more profound, noteworthy experiences from complex datasets. With AI analytics, businesses will not as it were see and get it what happened, but moreover why, how frequently, what may happen following, or what might have happened with a diverse course of activity.
As in all innovations based on machine learning, AI analytics gets more exact over time through learning and picking up more information within the handle.
The benefits of AI in analytics
Whereas able to say different points of interest of AI analytics, the application will have two major preferences over conventional information analytics: prescient experiences and robotization, each of which brings a few enhancements.
Predictive Insights
Expecting Patterns: AI-powered analytics can process vast volumes of historical and real-time data to identify patterns. By recognizing hidden trends, businesses can anticipate market shifts, consumer preferences, and emerging opportunities, allowing for in-time decision-making.
Improved Decision-Making: Predictive insights give decision-makers actionable information about potential outcomes so businesses can make informed choices to drive innovation and optimize resource allocation.
Optimized Showcasing Techniques: In marketing, AI analytics help forecast customer behavior and preferences. This knowledge is then used to create personalized campaigns and product recommendations that will better resonate with individual consumers.
Mechanization
Streamlined Forms: AI integration into analytics automates repetitive tasks, reduces the manual effort of data analysis, and accelerates the generation of insights, freeing up valuable human resources to focus on more strategic tasks․
Real-time Reactions: AI analytics facilitate real-time monitoring of data streams, leading to timely actions.
Productivity and Exactness: Automation minimizes the risk of human errors associated with manual data processing.
Fetched Reserve funds: Automation reduces operational costs by decreasing the need for manual labor while at the same time ensuring productivity and resource efficiency.
The Role of Data in AI Analytics
The core of analytics, be it traditional or AI analytics, is data; it is the essence and the foundational asset upon which we extract insights, see patterns, and make informed decisions. With new information gathered from data, we can transform raw information into knowledge used to drive progress.
What is data in analytics?
Data source: Information is the essential source of data that fills the analytics travel. It may be organized from databases, unstructured from social media channels, or semi-structured from emails.
Setting and understanding: Information could be an entryway that leads you to the understanding of complex scenarios. It may uncover the circumstances, inspirations, and factors and appear a bigger picture.
Designs and patterns: The most esteemed of information analytics is to appear designs and inconsistencies in datasets that offer assistance to construct optimization techniques.
Trade insights: Information analytics is crucial for data-driven choices, which is, in turn, crucial for commerce survival. In this light, information makes a difference and we get its forms and make educated choices.
Problem-solving: Information appears where the issue is, uncovers the root causes of issues, and makes a difference make in-time optimizations.
Advancement: Data-driven experiences are best to get its patterns (for case, in client behavior) and create items that cater to client needs.
What are the types of data?
Information comes in different shapes, depending on its source. Understanding the distinctive information sorts and knowing how to work with them is fundamental for viable examination and decision-making. AI analytics will work with all common sorts of information, counting the taking after:
Structured data
This sort is profoundly organized and effortlessly quantifiable, regularly put away in databases, and comprises lines and columns. Organized information is well-suited for conventional database administration frameworks and is commonly utilized in commerce operations and money related records.
Unstructured data
The sort needs a particular arrangement and doesn't fit flawlessly into columns and columns. It can be content, pictures, sound, video, social media posts, or emails. Unstructured information is wealthy in setting and frequently requires advanced devices like normal dialect preparation and computer vision to extricate meaning and experiences. AI analytics are idealized for preparing unstructured information.
Semi-structured data
Semi-structured information lies between organized and unstructured information and encompasses a certain degree of organization but doesn't accommodate to the inflexible structure of conventional databases. Illustrations incorporate JSON (JavaScript Protest Documentation), XML (eXtensible Markup Dialect), and NoSQL databases.
Temporal data
Transient information incorporates time-based data, extending from straightforward time stamps to occasion arrangements. This sort is fundamental for following changes over time, analyzing patterns, and making expectations based on chronicled designs.
Categorical data
Categorical information speaks to discrete categories or names without any inalienable arrangement. Illustrations incorporate sexual orientation, color, and item categories. Categorical information is regularly spoken to as ostensible or ordinal information, depending on whether there's a significant arrangement among the categories.
Numerical data
Numerical information comprises numbers and can be advanced categorized into nonstop and discrete information.
Leveraging AI for Insights
With AI, commerce insights has no more limitations, and it is now not a time-consuming and labor-intensive work to set up and collect information. Counterfeit insights, machine learning, and common dialect preparation have made BI more clever and basic in today's data-driven world.
Let's investigate which strategies contribute to significant bits of knowledge for comprehensive information investigation.
Machine Learning
Machine learning includes preparing calculations to recognize designs and make expectations based on information. In information analytics, ML plays a transformative part by working with expansive datasets, computerizing forms, and making forecasts. ML calculations recognize covered up designs and connections and reveal profitable bits of knowledge to be afterward utilized in decision-making.
Natural Language Processing
Whereas NLP is regularly related with innovations in connection with clients, in information analytics, characteristic dialect preparation makes a difference to extricate bits of knowledge from unstructured information such as pictures, writings, client surveys, social media posts, and reports. This innovation empowers opinion examination and can be utilized in social media observing.
Neural networks
Mimicking the human brain, neural systems have interconnected nodes (like neurons within the brain) that handle information to perform different errands such as design acknowledgment and classification. This subfield of machine learning is fundamental in information analytics for fathoming complex issues, learning from information, and recognizing connections. In reality, neural systems are capable of prescient analytics, design acknowledgment, and NLP.
Deep learning
A subset of machine learning includes the utilization of neural systems to handle large-scale and unstructured datasets, counting pictures and recordings.
How do AI Techniques Derive Actionable Insights?
Affirm, we have these methods, but how precisely do they offer assistance to pick up noteworthy bits of knowledge?
Pattern Recognition: Machine learning calculations naturally recognize designs in information that people might miss. With ML, human information investigators can get patterns, client behavior, and relationships that illuminate commerce choices.
Predictive Analytics: Machine learning models anticipate future results based on verifiable information, hence permitting us to afterward estimate requests, distinguish potential dangers, and/or optimize asset assignment.
Personalization: Through analyzing huge volumes of information, AI analytics uncovers client inclinations, behaviors, and intuitive to tailor encounters that can be utilized to construct personalized promoting campaigns, item suggestions, and upgraded client fulfillment.
Real-world examples of Insights gained with AI
E-commerce: With machine learning calculations, it is conceivable to analyze browsing and obtaining behavior and have item suggestions custom fitted to personal clients, expanding transformation rates.
Healthcare: AI empowers therapeutic records and quiet information examination to anticipate illness hazard, empowering early intercessions and personalized treatment plans.
Finance: Machine learning models can foresee stock cost developments by analyzing verifiable advertised information and variances, helping financial specialists in making educated choices.
Customer Service: NLP-powered chatbots handle client information and give moment reactions to inquiries, progressing client benefit.
Marketing: Opinion investigation of social media information makes a difference marketers get brand recognition and alter campaigns appropriately.
Data Collection and Preparation
When joining AI analytics into commerce forms, there are a few chronological steps to guarantee solid experiences from different sources.
Data collection
Data collection is the beginning arrangement that includes gathering data from different sources, such as databases, studies, sensors, and social media stages. It's pivotal to characterize the scope, reason, and criteria for information collection to guarantee that the proper information is gotten. With AI analytics, we decide the sources once and depend on machine learning to robotize the method of information collection.
Data discovery and profiling
After data collection and some time recently collecting and preparing the collected information, there's one pivotal step that makes a difference: the nature and structure of the information. Information revelation includes distinguishing information sources, their groups, and potential connections. Profiling incorporates evaluating information quality, distinguishing lost values, and recognizing holes that might impact investigation.
Data cleansing
Based on information disclosure and profiling, data cleansing will channel all the collected information, taking out irregularities and mistakes inside the dataset. This step, one more time, guarantees that the information is exact and dependable for ensuing examination. AI analytics will consequently input lost values, rectify typos, and expel copies.
Data structuring
When working with diverse sorts of information and data sources, it regularly arrives at completely different designs. Organizing includes organizing information into a steady and uniform organization, making it less demanding to prepare and analyze. This step guarantees that the information is prepared for significant bits of knowledge to be determined.
Data transformation and enrichment
Time to work with collected and cleared data by changing over it into a standardized organize, frequently appropriate for expository devices. This step might include amassing data, making calculated areas, and encoding categorical factors. Robotized information enhancement upgrades information quality by including pertinent data from outside sources and moving forward the setting for investigation.
Data normalization
The step includes organizing data to see comparatives over all areas whereas at the same time dispensing with redundancies and making strides keenly. Hence, it'll be conceivable to utilize the data for advance examination.
Data validation and publishing
At last, after handling, it is basic to approve that the data meets quality measures and is adjusted with the destinations of the examination. Once approved, the arranged data can be distributed or made accessible for advance preparation by human data investigators.
Challenges in data collection
Indeed in spite of the fact that the method is completely robotized and no human interaction is required, there are still challenges and issues which will require human insights.
- Data Heterogeneity: Assorted data sources may utilize distinctive designs, structures, and dialects, requiring endeavors to standardize them.
- Data Incompleteness: Lost or inadequate data can ruin investigation and lead to skewed bits of knowledge.
- Data Quality: AI analytics devices quicken data collection, but they may too influence information quality. Guaranteeing reliable quality over assorted information sources can be complex, as each source may have interesting issues.
How much does it cost to develop an AI app?
Using innovative AI analytics applications is a definite advantage over competitors because you have invaluable data at your fingertips. Meanwhile, developing your own AI application that can be used for internal purposes or shared with other users opens up a totally new perspective. In fact, investing in AI applications today can be the best business decision because AI is not the future; it is already the present.
The trending question, How much does it cost to develop an AI app?” doesn’t have a clear answer. The cost of an AI app may vary from $50,000 to $150,000 depending on the project type, software type, features included, hardware, and development rates.
Factors affecting the AI app development cost
Project type: Depending on whether it is an app from scratch or a customized one on a pre-made model, the development price will radically differ. A custom-made app with unique features will cost a lot, but at the same time, it will be more valuable.
Software type: Depending on the application type, it will have a set of must-have features that also affect project cost. AI applications can be NLP and ML, deep learning, machine vision, voice and face recognition with Artificial narrow intelligence, etc.
Hardware or cloud: The price may change depending on which option is chosen to store data. Hardware is more expensive based on its computational power and storage capacity. At the same time, cloud services may charge extra for training ML or processing large amounts of data.
Expertise: The project’s success greatly depends (if not completely) on the development team’s expertise. If it is an offshore agency, the criteria for partnership should first be based on previous experience with similar projects.
Of course, there are a million more aspects affecting the final cost of AI app development that are discussed with business analysts.
Building AI Models
With everything said and talked about, it is time to induce the viable prepare, i.e., building an AI show custom fitted for analytics. As true professionals in our field, we'll actualize all steps, beginning with cautious arranging up to arrangement.
Problem Definition
The travel starts with a clear understanding of the issue and the particular objectives of the AI show. This incorporates characterizing the scope, targets, and sorts of bits of knowledge.
Data Preparation
Data preparation is the key to building precise AI models. As of now specified, information collection goes through data cleaning, change, and organizing.
Algorithm Selection
Choosing the proper calculation is the perfect way to guarantee the victory of an AI demonstration. Depending on the nature of the issue, the sort of information, and the specified result, distinctive calculations will exceed expectations in different scenarios.
Training the Model
Preparing an AI show is another noteworthy organism that involves exposing it to the arranged information and permitting it to memorize patterns and connections. Machine learning gets more brilliant with learning, so the work here is to supply it with the essential preparation. Amid preparing, the show alters its inside parameters to play down the contrast between its forecasts and real results.
Validation and Testing
Approving the model's execution is vital to guaranteeing its unwavering quality. Data that the demonstration hasn't seen amid preparing is utilized to assess its precision, exactness, review, and other execution measurements. At this organization, it'll be clear whether the demonstration performs similarly well on the preparing information and the modern one.
Model Optimization
Further iterations after introductory approval will optimize the model's execution. This might include altering parameters and fine-tuning calculations.
Deployment and Monitoring
Finally, when an AI model meets the desired performance standards, it's deployed in a real-world environment. Constant monitoring will help ensure that the model's predictions remain accurate over time.
Implementing Real-time Analytics
Today’s fast-paced trade environment ought to be followed with capable advances in real-time. And that’s where real-time information analytics enters the diversion. AI is perfect for giving a full range of real-time information collection and examination highlights that bring different benefits.
Timely insights: Real-time analytics back up-to-the-minute experiences, empowering following openings and tending to challenges instantly.
Improved decision-making: With real-time bits of knowledge, decision-makers can base their activities on the foremost current and significant information, in this way making more precise and compelling choices.
Enhanced customer experience: With real-time analytics, client benefit operators can react to client needs in real-time, personalizing intuitive and making strides client fulfillment.
Agile operations: Real-time information empowers changing operations and methodologies rapidly based on conditions and optimizing effectiveness.
Visualization and Reporting
One of the challenges of AI analytics and BI is the visualization of information in a way that creates it simply to get it. In a perfect world, information ought to be intuitively, and engaging. That’s why visualization may be a noteworthy component in information analytics since it changes complex information into effortlessly justifiable and shrewd visual representations.
In AI analytics, machine learning and counterfeit insights progress information visualization through information expansion, common dialect inquiries, and explanations. It bridges the crevice between crude information and noteworthy bits of knowledge, permitting information investigators to encourage work with it to see designs, patterns, and connections.
Role of Visualization in AI Analytics
Other than the self-evident advantage of giving a clear picture of the information, visualization is vital for the taking after reasons:
Simplification: Complex information is changed into visual designs that are simpler to get and decipher, indeed for non-technical gatherings of people.
Pattern Recognition: Visualizations offer assistance clients distinguish designs, patterns, and exceptions that might go unnoticed in raw data.
Comparison: Visual comparisons between two or more information focuses or categories make it much simpler to urge a fast understanding of varieties and connections.
Storytelling: Visualizations are utilized for making accounts that direct clients through information and bolster the communication of bits of knowledge.
Decision-Making: Clear visualizations enable decision-makers to form educated choices based on a clear and comprehensive understanding of information.
Visualization Tools and Techniques
At Addevice, we utilize as it were the most recent innovations to get a handle on real-time information, get information visualization, and pass on vital bits of knowledge.
Data Visualization Libraries: Depending on the programming dialect, there are different information visualization libraries to form visualizations. Highcharts, Toast UI Chart, and D3.js are prevalent JavaScript information visualization libraries. The best Python libraries are NumPy, Pandas, and Matplotlib.
Interactive Dashboards: Devices like Scene and Control BI permit for the advancement of intelligently dashboards to investigate information powerfully.
Custom Visualization: Tailor-made visualizations are outlined to address particular business needs and show information within the most important and impactful way.
Examples of data visualization dashboards
Collected, handled, and visualized information is sent to information visualization dashboards with intuitively graphical interfacing that permit partners (marketers, information investigators, and information researchers) to track information, information sources, measurements, and patterns from a dataset. Information visualization dashboards are broadly utilized over businesses for different purposes, counting:
Digital marketing dashboard
This dashboard shows key execution pointers (KPIs) such as click-through rates, transformation rates, and return on speculation (ROI) for distinctive advertising campaigns. Intelligently visuals permit partners to investigate information over campaigns, stages, and socioeconomics.
Social media analytics dashboard
A social media analytics dashboard shows measurements such as devotee development, engagement rates, post reach, and estimation investigation. Visualizations incorporate engagement patterns, top-performing substance, etc.
E-commerce analytics dashboard
An e-commerce dashboard gives bits of knowledge into online deals, item execution, client socioeconomics, behavior, and shopping cart deserting rates. It may incorporate information on top-selling items, client surveys, and topographical deals conveyance.
Challenges and Future Directions
AI is colossal. It is the unused cash of the computerized environment, which offers a competitive advantage in any case of the industry. Still, is everything coming full circle? Are there any pitfalls to AI? What are the challenges of AI analytics? Is it time to switch from ordinary exchange bits of knowledge to AI?
Here are a couple of common challenges you may stand up to with AI analytics.
Data Quality and Quantity: The accessibility of high-quality and adequate information for preparing AI models can be challenging. Fragmented or one-sided information can lead to wrong experiences and forecasts.
Model Complexity: Creating complex AI analytics applications requires skill in machine learning and profound learning.
Interpretable Insights: AI models ended up more complex, and clarifying the thinking behind their forecasts can be troublesome.
Data Security and Privacy: The most basic and trending issue in AI applications is taking care of touchy information whereas keeping up security and security.
Infrastructure and Resources: AI analytics requests significant computational power and foundation. The biggest challenge is scaling up the foundation to handle expansive datasets and complex models.
Cost and ROI: While AI applications have remarkable execution and a better ROI, they are costly and still require restricted ability to construct and keep up with them.
The future of AI analytics: Conclusion
The potential of AI and AI analytics is boundless. In fact in its starting with days of life, AI analytics will enlist higher execution and capability and give much predominant comes about over time. In this article, we endeavored to reveal the center shapes of AI analytics, its role in exchange shapes, and the importance of contributing in AI applications these days. As a foot line, we would like to share the creating designs in AI analytics we are going some time recently long be executing:
Explainable AI (XAI): The incline towards sensible AI centers on making AI models more clear and justifiable. Usually frequently particularly imperative in healthcare.
Automated Machine Learning: AutoML stages will come forward to improve the strategy of building and preparing AI models and make AI more open to a more broad gathering of individuals with moving levels of capacity.
AI-Powered Natural Language Processing: While the development is no longer an headway, it'll continue advancing, enabling AI to induce it and create human tongue more suitably.
Read also The Role of Ai in Healthcare.
AI Analytics App, Make a Chatbot, Uber Like App Development, Analytics App, Apps Like Siri, Taxi App, Software Development, AI App, Delivery App, Clutch, ML App, App Development, Booking App, IoT App, Uber App, Wallet App, Dating App,
Get an instant AI app development
Embark on the journey of building your AI analytics app from the ground up.
Our Approach Includes:
✅ In-depth AI analytics strategy
✅ Customized development solutions
✅ Proven success in AI app development
Table of contents
FAQ
An AI analytics application gives a solid competitive advantage to businesses that need to use information for educated choices. For Addevice.io, AI analytics empowers optimizing app execution, upgrading client encounters, and making vital choices based on data-driven experiences.
An AI analytics app can collect measurements from diverse sources and for diverse dashboards, counting client intuitive, app utilization measurements, gadget data, and more. This assorted information pool permits Addevice to get its client behavior, recognize patterns, and make data-backed advancements to its administrations.
Not at all like conventional analytics apparatuses, an AI analytics app is based on machine learning calculations that distinguish complex designs that are some of the time unnoticeable to people. AI analytics donate more exact expectations, personalized proposals, and the capacity to handle tremendous sums of information.
Real-time analytics include preparing and analyzing information as it's created, giving moment experiences. At Addevice.io, we keep up with the pace by gathering and analyzing information in real-time hence having up-to-date information approximately our accomplices and their needs.
The most common challenges of building and keeping up AI analytics applications are information quality confirmation, selecting the proper calculations, overseeing computational assets, and guaranteeing protection and security. Tending to these challenges requires a devoted group with important skill and nonstop advancement endeavors.